March 26, 2024
Mastering xG Statistics for Football Prediction Success
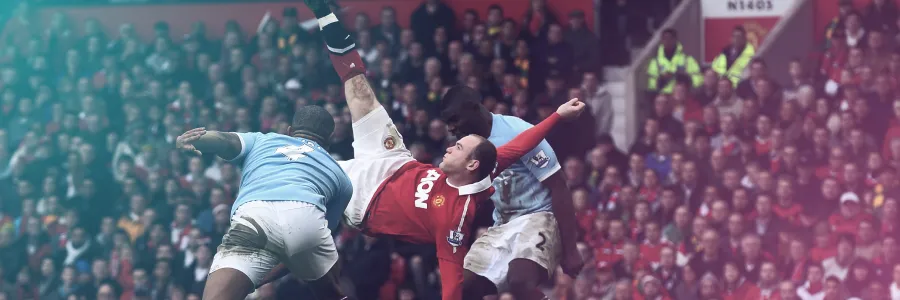
Expected Goals, or xG, first evolved as a notion during the 2012 MIT Sloan Sports Analytics Conference. This concept was designed for ice hockey to evaluate performance and scoring. The developer, MIT undergraduate Brian Macdonald, used it to analyse Premier League goal scorers. It has matured into a working football match analysis model. A model from Opta Sports predicts a team's goal score in their next encounter. This indicator helps determine if a team performed below or over expectations based on results.
xG (Expected Goals) is a metric that uses various elements to calculate and determine a team's goal-scoring potential. After multiple updates, the model now accounts for shot quality, shot height, goaltender position, and the team's attacking and defensive strengths. Shot probabilities range from 0.01 to 1. Consider a 0.25xG probability shot. This gives the shot a 25% chance of scoring.
We can anticipate a team's match performance using xG's numerous models. Multiple analysis is necessary to produce accurate football forecasts. This platform lets you anticipate football matches using xG data. The site's expert football betting advice might reduce your losses. Welcome to xG football calculations. We'll explain this statistic, how to utilize it in projections, and its pros and cons.
Exploring the intricacies of xG calculations
A vast photo database was used to test and evaluate xG. Shots from any position can be assessed and predicted to score. The model can now assess probabilities for more factors based on shot strength. Using xG to forecast goals from shots involves many parameters. A game shot must be evaluated using numerous factors. These factors include whether the shot was a set piece or open play, the shoot opportunity, shot quality, placement, and angle.
The model shows that near shots are more likely to score than long shots. In-depth xG models consider the opponent's attacking and defensive capabilities. These parameters allow xG to predict the home club's goals based on their attacking ability, the away team's defense, and the league average. This approach helps predict away team goals. One must evaluate the away team's attacking and defensive strengths and the league's average away goal score when predicting goals.
Over the years, xG has been modified to calculate probability other than shot goals. There are many sports analyzing metrics. These markers are vital to team success. These metrics include xG/90, which measures expected goals for 90 minutes played, npxG, which focuses on non-penalty expected goals, xGf, which calculates expected goals for matches after they've been played, xGa, which estimates expected goals conceded, xA, which evaluates expected goal assists, and xPts, which predicts a team's expected points.
Integrating xG Into Predictions
XG models may help make smart football bets. Consider how you've utilized the metric to analyze a team's regular matches. This lets you see if they meet expectations. As matches develop, xG statistics can help you predict. With its ability and past data analysis, xG leaves little to chance.
Fortunately, the several xG versions let you thoroughly analyze a team before wagering. These assessments evaluate teams' playing qualities, predicted goals conceded, and open play opportunities.
Exploring the advantages and drawbacks of utilizing xG
The introduction of xG gave football new statistical insights. Its ability to analyze teams' past and present statistics shows how well they create chances and shots. This report includes team chances, missed opportunities, and goals conceded.
Developers are working on advanced and precise xG statistics. These data will include xG fairness, luckiness, anticipated goals on target, by halves, and predictability. To determine if a team's win or loss was due to skill or luck, fairness and chance must be considered. Soccer statistics like shots on goal and projected goals per half are easy to grasp. In addition, xG predictability measures how well the model's predicted outcomes match the actual scores. XG reduces prediction uncertainty with its plethora of facts.
This paradigm has benefits, but its drawbacks must be acknowledged. This metric cannot account for team spirit, morale, or weather during a match. Injury and referee bias must be considered in addition to the game. Several factors can affect game tempo, making xG's estimates inaccurate.
One must also evaluate each player's shooting, attacking, and defending skills. Motivation during a game might affect the team's chances of winning. Football matches are continually changing, therefore an xG statistic cannot capture all the complexity. Future advances can provide an analytical model that captures these abstract components.
In conclusion
Football statistical analysis was transformed by xG statistics. These variables help predict a match's outcome, reducing forecast uncertainty. Since other abstract elements have yet to be examined, the key metric needs more development. We advocate using the xG metric and its updates to optimize football betting success.
- Best xGscore predictions
- Profitability ROI 7% and higher
- 60+ bets per month